Proptech Partners With Universities to Solve Real Estate Challenges
Columbia's AI center among the vanguard of schools working with developers, lenders and tenants
By Philip Russo January 31, 2023 9:00 am
reprints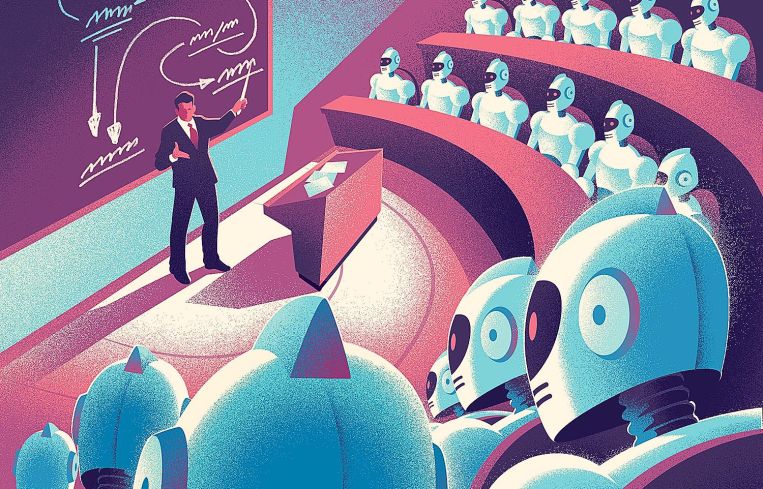
The next step in the development of technology solutions for some of real estate’s most complex issues may not be happening in the offices of developers or at proptech startups. Instead, it might be happening in the halls of academia previously little associated with the world’s largest industry.
One such academic institution working to apply sophisticated technology to real estate challenges is Columbia University. There, Josh Panknin leads real estate, artificial intelligence (AI) research and innovation at The Center for Artificial Intelligence in Business Analytics and FinTech, part of Columbia’s Fu Foundation School of Engineering and Applied Science.
Panknin, who has an academic and professional background in finance, financial technology and real estate, including stints with Ackman-Ziff and Deutsche Bank, is among the current cohort of academics partnering with traditional real estate developers to solve complex issues through the use of AI and applied data science.
While proptech has done much to integrate technology into real estate, it is a narrow first step toward the sophisticated use of tech by the industry, said Panknin. Corporations’ difficulties in integrating technology into their businesses have long exasperated data scientists and quantitative analysts from the financial and academic worlds, regardless of the industry. One result of that frustration was the formal creation of Columbia’s AI Center in May 2022, Panknin said.
“We were all very frustrated that there were so many opportunities out there to either address problems and inefficiencies or to develop technology,” he said. “The typical methods, the things we’ve been using for the last 20 to 50 years, have become pretty efficient. The only way to get more efficient is to develop and apply new tools to old problems.”
As Columbia boasts some of the “best technologists in the world in machine learning, deep learning experience, and quantitative backgrounds,” Panknin said, the center set out to learn the real estate industry’s problems, engage with companies, and let its students use the academic environment to solve them. “But it’s not theoretical. It’s not just an academic exercise. We’re building with the vision of creating software and developing tools that we can take back to these companies and let them use in the real world that is more complex.”
Among the other universities addressing real estate technology development are the Massachusetts Institute of Technology and New York University. Some of the tools Manhattan-based Columbia’s AI center brings to clients are the fundamental and advanced application of data science, including AI, Panknin said.
“Machines solve problems in very different ways than humans do,” he said. “Most people think that taking the same data humans use to solve problems and giving it to a computer to use will result in ‘AI’ giving a better answer. That’s 100 percent incorrect.
“Most real estate companies don’t understand that the analytics process, or any tech process, involves a sophisticated pipeline of ideation in a machine-suitable way: data collection, cleaning, processing, storage, building learning algorithms, and then using models in production. Most of these processes sound boring and too in the weeds, but without them you have zero chance of success using data and analytics.”
One big problem: The current proptech environment is full of hype and unrealistic claims about what technology can do. Panknin said this leads to unrealistic expectations from real estate companies and results in numerous failed projects.
To educate the real estate industry, the center has an initiative around developing an open-source database for real estate-related data that is ready for AI/machine learning applications. “The idea here is that most real estate companies can’t get value from data scientists because they don’t have enough data to work with,” Panknin said. “And most real estate companies aren’t going to pay for data scientists to clean data for two years before they start seeing a payoff from them. So by forming this database, real estate companies can more quickly get value from data scientists and engineers.”
Among the center’s clients are Deloitte, JPMorgan and RXR, all of which have deep financial interests in real estate development and investment. Panknin leads the projects for the companies. The AI center has been working with RXR and JPMorgan since June 2020, and with Deloitte since September 2021.
One of Panknin’s main jobs is to be what he described as the translator between the technologists and real estate professionals. “There is a gap between them that makes effective communication nonexistent,” he said. “You cannot bring a real estate person together with a technical person and solve complex problems well. You have to have translators in the middle.”
The center, for example, is working with RXR on understanding how and why individual neighborhoods within metropolitan areas often move at sometimes drastically different levels of price appreciation.
“The goal was to partner Columbia with our in-house team that’s working on this full time,” said Andrew Min, senior vice president of strategy and digital initiatives at RXR. “How can we find an academic partner that can bring to bear the latest and greatest that academia understands in terms of advances in data science, new algorithms and new approaches, as well as partner with students that live this full time, and help them understand the practical side of data science, statistics and machine learning?”
RXR’s thesis is that data can and will be used increasingly by the commercial real estate industry to change everything, said Min. “Specifically the areas we’re excited about are making better investment decisions, and using data to inform how to operate our buildings better and ultimately to create better customer experiences.”
Data engineering and advances in data science as an academic discipline, along with the explosion in the amount of data sources, has led to similar changes in retail, hospitality, and essentially in every other industry outside of real estate, he added. “We think those same forces will operate in real estate as well.”
The RXR project uses around three to five Columbia graduate data science students, and is focused on the near and long term, said Min.
“The neighborhood growth model is still ongoing,” he said. “That’s one that probably will still take some time, but we try to have clear deliverables at the end of each semester. So, today, what we’ve done is we’ve analyzed a lot of different data sources — traditional economic data, but also things like building permits, 311 calls, Yelp data, transit patterns and demographics. We’ve also done a lot of work on data architecture and engineering. What’s the sensible way to connect all this data together and store it in a way that’s usable and scalable?”
It’s not all academic, of course. RXR is a successful real estate developer and owner, and wants any project results to further that success.
“We’ve also started building shorter-term products that our investments team can use,” Min said. “For example, we built a platform where they can see places of interest as described by Yelp, overlaid around our various investments. You can see bars, coffee shops, parks or other types of places of interest near our given investments, and you can better understand their character.”
In addition, RXR tries to make the students’ experience working with the company a rich one, said Yoann Poirier, lead data scientist at RXR.
“We tried to focus on what the students are going to get from the project,” said Poirier. “Even though the project is going for multiple years, we’re trying to get a reward at the end of a semester by making sure that the students get some knowledge from that. Running a project like that is not always fun. There’s a lot of data cleaning, for instance, when we use public data. This is something that can be valuable for the students, but sometimes not so much, because it can be a lot of work.
“So we want to make sure that they gain the most out of it by also working on the data engineering, as well as data science, and studying building predictive models. Even though we’re not comfortable to actually say, ‘We finished a predictive model that has been moved to a prediction,’ we’ll make sure the students actually gain some knowledge in the process.”
Philip Russo can be reached at prusso@commercialobserver.com.