Cherre CTO Ron Bekkerman On ‘Owner Unmasking’
The company and its top data scientist seek to build a trove of information for property investors to mine
By Philip Russo October 26, 2021 8:00 am
reprints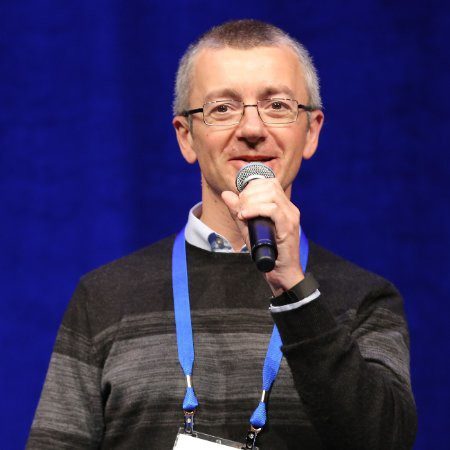
Three years ago, Ron Bekkerman didn’t know anything about the real estate industry. However, armed with a bachelor’s and a master’s from Technion — Israel Institute of Technology — and a PhD in computer science from the University of Massachusetts at Amherst, he was hired as chief technology officer for Cherre, responsible for “building the world’s largest real estate knowledge graph,” as the proptech startup claims.
Proptech Insider spoke with Bekkerman in mid-October about leading Cherre’s ambitious tech platform and how he became “the number four data scientist in the world.”
The interview has been edited for length and clarity.
PropTech Insider: How long have you been with Cherre, and what are your duties as CTO?
Ron Bekkerman: I’ve been here for three years. The company is about five years old, so when I joined, the company was very small. I experienced the growth of the company from a small size to where we are right now, about 80 people, and we are going to grow even more.
It’s a very interesting question, what the CTO of the company is supposed to do to start with. In many cases, the CTO is actually the chief engineer, the person who is responsible for all the engineering development in the company. In many startups and many companies, there is a split of those roles: the VP of engineering, the person who actually is responsible for the day-to-day engineering development; and the CTO, who is mostly responsible for the future direction and vision for where the company is supposed to be in two, three, five years.
If a [CTO] is not out there like a North Star, we are going to all be stuck in engineering. This is very scary for a startup because it needs to develop to go forward. It’s not like IBM, where you do whatever you’ve been doing the last 70 years, otherwise everything is going to fall apart.
Startups are completely different. I’m very fortunate to be in this position to be responsible for everything that we’re going to be doing in the future, which obviously includes analytics, data science, AI and all the terminology that we usually use nowadays.
I’m very biased. I was teaching data science before I came to Cherre, so I need to start with definitions, because otherwise my students wouldn’t know what I’m talking about. I love that.
Where did you teach data science?
My first 20 years in Russia, nine years in Israel, nine in the States, six in Israel, [another] three in the States. So Cherre hired me directly from Haifa, where I was teaching in the University of Haifa.
How do you deal with essentially holding two jobs as Cherre’s CTO?
First of all, I must admit that it’s not easy. When you come to a small company, in a very senior role, my fear was that people would ask, ‘Why did we hire this very senior guy? We don’t really know what a senior CTO is supposed to do.’ So I needed to be productive right away.
I was the first, the only, data scientist and, for much of the first year, I was sitting in the corner writing code. To start with I was a pretty risky [choice], because I had been a professor for five years and my students would be writing code for me; but for those years I actually was running a startup, so I was developing technology. I wasn’t absolutely hands-off. So I built the first level of Cherre’s technology. Now we’ve hired a team, but to start I was pretty much all by myself doing this grunt work. If I wouldn’t do that, no one would.
At this point how many people are working under you?
Four people, three in the States and one in Israel.
Cherre’s platform does many things. As CTO, what’s your mission?
We are in the data aggregation business. My team and I are responsible for cleaning all this data. The most interesting work starts when we can have access to all this data, cleaned and integrated together. We spent three years cleaning data. It was in our DNA to start with. Now, we’re integrating data and we have this ability to do analytics that we couldn’t do before we have clean integrated data.
We have many projects popping up that are exciting. We can do stuff that no one in the industry can do, because we are seeing all this real estate data in one place integrated and cleaned by ourselves. Real estate data is very dorky. I’ve seen many different data sets over the last 25 years. Real estate data is the dorkiest probably out of all them. It’s very hard to work with before you clean the data.
Do you get your data from landlords, tenants, others? What do they want you to do with the data, because there is always the question of ‘how do we monetize this?’
It comes from many sources — public, private, and third-party data. We’re integrating all of them. The private data is the most interesting part actually. Some of our customers give us their data, because they have trouble integrating their own data. Bigger organizations have so many diverse data sources, but they don’t know how to monetize their own data. They’re giving us their data. We clean it, we integrate it with all of what we’re seeing, and we’re returning this data back to them in a clean format exactly for their analytics.
But what we are doing with this data is owner unmasking. As you know, it’s very hard to figure out who the commercial owner is for many different properties, for many different reasons. So we are unmasking the owners on scale, meaning that we’re focusing on every, single owner in the U.S. It’s not like RCA [Real Capital Analytics] that cares about only the biggest ones. We are saying we need to amass the ownership for every, single property. I can’t say that we are doing it perfectly. What we care about the most is the office building that costs $20 million, somewhere in a city in the United States. Who owns that? That’s what our system does.
This is the foundation of our technology that we invested a lot of time and effort in building. That’s what is providing us visibility [into the market] and many other abilities that are the knowledge graph I’m very very proud of.
Does Cherre get data from residential as well as commercial projects?
Everything. We really care about not separating residential and commercial. First, because many commercial investors are really interested in residential properties as of now. Second, if you only focus on commercial or residential, it’s mixed in geography. You have a single-family residence and maybe a gas station next to it. The gas station really affects the price, the quality of the air and many things that are related to this single-family residence. If you separate them — don’t take the gas station into account — you’re totally doomed.
There are so many cases like that, not only in suburban areas but the city as well. This needs to be taken in a holistic way, and that’s what Cherre is all about.
How else does Cherre use this aggregated data?
We can do portfolio analytics. We can say what’s going on with every owner right now, what the owner owns.
What we don’t have yet is historical trends or unmasking historical transactions. Once we have done this, we can build a timeline of what is happening with a specific owner. Are they buying, selling, moving from one market to another, or moving from one asset class to another? Right now, we are sitting on a list of 100,000 commercial owners; and scale here really matters because then we can say we can do predictions; we know that this is what the investor is currently buying, this asset is currently selling, that this investor would be interested in this specific property given the analysis we did on their portfolio.
Obviously, this is a very far, stretching goal, but it’s not only theory. We can potentially build the off-market market. We definitely need to partner with many organizations, but, if we manage to do this, just think about what can happen in commercial real estate. It’s going to be substantially more liquid. Think about the stock market, with trillions of dollars moving back and forth within milliseconds. In commercial real estate, you’re doing the deal, the due diligence, it’s half a year. You cannot buy and sell a $100 million property within a millisecond. That’s not going to happen. But, essentially Cherre really wants to help investors, because the market is just too slow.
What did you know about real estate before you became CTO at Cherre?
I’m not a businessman. So, when [an Israeli investor] introduced me to L.D. [Salmanson, CEO of Cherre] we exchanged our decks. I sent him my slides and he sent me his files and it was the same idea. Totally the same idea. So, I came to [New York City] to visit him and seven meetings later, I was like, ‘OK the business is here.’
If you could compare Cherre to another company, what company would you aspire to be?
Well, that’s a question for L.D., but, if you want my personal opinion, maybe the Palantir of real estate: a company that does a lot of very big data analysis and comes up with lots of insights that help many industries to move forward.
Data science is relatively new in real estate. What is the biggest obstacle you had to overcome in trying to do your job at Cherre?
As I talked about, the data is very dirty. [Real estate] started collecting in the `80s, and, back then, everything was one big typo. This is one of the biggest troubles. One of the questions that I really love asking is how many ways do you think someone can write JPMorgan [Chase]? Twenty-five? Try 25,000. That’s all the subsidiaries, divisions, branches of Chase Bank, with the name of the town, without the name of the town. We really need to aggregate it all together into one, because if we don’t we will have 25,000 different brands.
That sounds like this is the kind of job that can make you insane.
To an extent, but I just have a lot of experience with stuff like that. Some people can’t stand it. I’ve been doing it for years, and I know how to do it. I also hired a good team that helps me. It’s not just me dealing with it.
But another problem is talent. Data scientists are not really excited about working for real estate, because, again, the data is not clean and they don’t like cleaning data. They want to work with already cleaned data. Second, if you think about it, there is not that much data [in real estate].
We are talking about an order of magnitude of a terabyte of data that we’re dealing with. But, if you go to, say, advertising, it’s like a few orders of magnitude more data, so it’s more fun for them. And the data is cleaner to an extent.
Another thing is that they want to focus on those domains that have already been explored by many data scientists, like in the medical or finance fields. Real estate is like an enigma for them and they don’t really want to learn it because, ‘Why should I? I’m the data scientist who is getting a half a million dollar salary a year. I can work wherever I want.’ It’s not supposed to be like that, but this is the market as of now that we’re dealing with.
You like to tell a story about how you became the fourth data scientist in the world.
I joined LinkedIn in 2009 as a research scientist. I was one of the very first research scientists then with LinkedIn. One day, our manager came to our research scientists’ meeting and he said: ‘I don’t want to call you research scientists anymore. I’ll call you data scientists now.’ And I was like, what? It turns out that the guy actually created this term with another guy from Cloudera, whose name I don’t remember. He coined this term and he started marketing this new profession.
After he left, he became the chief data officer [of the United States Office of Science and Technology Policy]. His name is D.J. Patil. Because of that, I’m actually ‘data scientist number four’ in the world.
Back then I wasn’t happy about that at all. I was like, ‘I’m a research scientist. What is a data scientist?’ Now I’m very proud of the fact that I’m one of the very first data scientists in the world.Philip Russo can be reached at prusso@commercialobserver.com.